The initial algorithm derived from the foundational research in Basel was further optimized with data from the LURIC study.
- •The optimized algorithm was then broadly validated in real-world in- and outpatient settings with a female representation gap of only 3.7%.
- •Origin and Quality: Data was sourced from multiple high-quality clinical studies.
- •Data Preprocessing and Transformations:The preprocessing steps, including handling of missing data and data transformations, are well documented. For example, missing values were replaced with median values or constants within normal ranges.
- •Bias Investigation and Documentation: The studies utilized robust statistical methods and sensitivity analyses to ensure the stability and reliability of the models. The comprehensive analysis indicates a thorough investigation of biases.
The Cardio Explorer algorithm requires only easily accessible patient data from a set of clinical and laboratory variables.
Among these variables, sex (gender) is explicitly listed as one of the inputs.
- •Clinical Variables:Age, sex, weight, height, presence and type of chest pain, diabetes, nicotine use, pathological Q-waves (at ECG), systolic and diastolic blood pressure, and relevant medication like statin use.
- •Laboratory Variables:Mean corpuscular hemoglobin concentration (MCHC), white blood cells, urea, uric acid, troponin, glucose, total cholesterol, low-density lipoprotein cholesterol, high-density lipoprotein cholesterol, alanine aminotransferase (ALAT), alkaline phosphatase, amylase, total protein, albumin, and bilirubin.
The diagnostic accuracy of the tool is evaluated using the Area Under the Curve (AUC) from receiver operating characteristic (ROC) analysis. The AUC value ranges from 0.5, which reflects random chance, to 1.0, representing perfect diagnostic accuracy.
In clinical use, the Cardio Explorer algorithm demonstrates robust and strong diagnostic performance in predicting the presence of obstructive CAD:
- In high-prevalence CAD settings (inpatient setting): The AUC is 0.87, indicating the model accurately identifies obstructive CAD in 87% of cases. This level of accuracy is considered high and clinically significant.
- In low-prevalence CAD settings (outpatient setting): The AUC remains 0.87 overall, reflecting a strong and robust diagnostic performance also in lower prevalence settings.
These results underscore the tool’s consistent diagnostic accuracy across different clinical settings and patient populations.
Additionally, Cardio Explorer® has been validated for its ability to detect myocardial ischemia, as measured by PET imaging, with an AUC of 0.76. Interestingly, its performance is even better in female patients (AUC 0.77).
The slightly lower AUC in ischemia detection is attributable to the fact that the algorithm was specifically trained and validated to identify the anatomical presence of coronary artery disease (CAD) defined as >50% stenosis via invasive coronary angiography, rather than ischemia itself.
The algorithm was validated in four independent cohorts: Validation sample from the LURIC cohort (494 patients, with 33% female), a simulated low-prevalence population (30’303 patients, with 43% female), an independent outpatient cohort (696 patients, with 51% female) and a validation cohort for myocardial ischemia (2417 patients, 32% female). Overall, the validation data distribution consists of 42.3% female and thus, closely reflecting the general population's gender distribution.
- •Origin and Quality: Both validation datasets were sourced from high-quality studies in outpatient and diagnostic settings, enhancing the reliability of the algorithm’s validation results.
- •Bias Investigation: The validation was conducted with thorough statistical analyses, ensuring the algorithm's performance remained unbiased across gender and risk categories.
Level of evidence for AI validation: The algorithm was validated using external dataset, with performance metrics such as the area under the receiver operating characteristic curve (AUC) reported.
Results and Sensitivity Analysis: The results of the algorithm prediction were compared to established diagnostic procedures. The tool remains a highly valuable, evidence-based, non-invasive method for diagnosis and exclusion of obstructive coronary artery disease and myocardial ischemia.
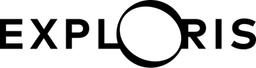