PIPRA
Preoperative risk assessment tool, to assess the risk of a patient developing postoperative delirium. The test uses standard medical data and provides an immediate score-based risk assessment.
Company:
Indications
Men
Women
Prevalence in Population
Men
18%
Women
18%
Gender Distribution of Population
Men
51%
Women
49%
Study Participation
Men
52%
Women
48%
Representation Gap
1%
Training Data Distribution
Men
54%
Women
46%
Representation Gap
3%
Validation Data Distribution
Men
55%
Women
45%
Representation Gap
4%
Accuracy
Men
90%
Women
90%
Study Representation
Common
The study used as basis for development includes data for 2,250 patients, with a gender distribution of 54.6% male and 45.4% female. This indicates a balanced representation of genders. In the subset of patients who developed delirium, 52.3% were male and 47.7% were female. The gender distribution in the foundational research only has a minimal representation gap.
Training Data Quality
Common
Gender Representation: As noted above, the training data includes 54.6% male and 45.4% female participants, which is balanced compared to the prevalence in the population. - Origin and Quality of Training Data: The article details that the data were sourced from eight high-quality studies (randomized control trials and cohort studies) - Transparency in Data Preprocessing: The study describes the preprocessing steps, including the handling of missing data and standardization procedures. - Investigation and Documentation of Biases: The study used the Quality In Prognosis Studies (QUIPS) tool to evaluate the risk of bias in several domains, including study participation, attrition, exposure measurement, outcome measurement, and study confounding. This comprehensive bias assessment indicates thorough investigation and documentation of potential biases and their impacts.
Validation Data Quality
Common
PIPRA was externally validated using two datasets from independent sources: Bern Dataset (Switzerland): Data were prospectively collected from patients undergoing surgery at a 70-bed Orthopaedic Surgery and Traumatology Department at the University Hospital of Bern. Patients were systematically assessed for postoperative delirium (POD) using the Delirium Observational Screening Scale (DOSS). This dataset provided robust, systematically collected data from a clinical environment, ensuring high external validity. LMU Dataset (Germany): A second dataset was collected from a study conducted at the Ludwig Maximilian University of Munich. Here, the 4AT Tool (and CAM-ICU for intubated patients) was used to assess POD in surgical patients. This data, although preliminary due to the ongoing nature of the study, contributes to a broader, real-world validation of PIPRA's predictive power. The external datasets were collected using validated, systematic methods for diagnosing POD, ensuring consistency and reliability in the outcomes. Handling of Missing Data: For the Bern dataset, missing variables such as ASA scores and CRP values were imputed based on predictor averages, minimizing data loss and maintaining model robustness without compromising the validity of the results. Diversity and Real-world Applicability: Both validation datasets were collected in diverse surgical settings, from orthopaedic to general surgery, enhancing the algorithm's applicability across various clinical environments. The systematic approach to collecting POD data and the high level of control over patient assessments ensures that the findings can be generalized to other settings where PIPRA might be used.
Algorithm Adaptability
Common
The PIPRA algorithm explicitly excludes gender as an input variable because the development it was determined that it is not a significant factor in predicting postoperative delirium. While this decision is based on the algorithm's performance and the analysis conducted during its development, it indicates that gender-specific needs were assessed and found not to be a determining factor for this particular prediction task. This approach reflects a data-driven decision to focus on more impactful variables, even though it means gender-specific adaptability is not directly addressed in the model.
Accuracy
Common
The PIPRA algorithm explicitly excludes gender as an input variable because the development it was determined that it is not a significant factor in predicting postoperative delirium. Therefore, the accuracy is identical for men and women. The algorithm has a high sensitivity of 90% for high-risk patients (meaning it correctly identifies 90% of patients who will experience delirium). This high sensitivity indicates that the algorithm is effective at catching most cases of delirium and therefore has a low likelihood of missing patients at risk. For patients classified as low risk by the algorithm, there is an 86% to 95% probability that they will not experience delirium. This high probability suggests that the algorithm is reliable in identifying patients who are genuinely at low risk.
Transparency
Common
The PIPRA provides details on the hyperparameters (logistic regression with an L1 penalty), the training dataset (data from eight studies), and the training process (10-fold cross-validation for predictor selection and internal validation).
Accessibility
Common
The PIPRA algorithm's user interface is designed for clinical use, providing meaningful output of absolute risk percentages and the importance of variables. The documentation enables the healthcare professional to use PIPRA.
Affordability
Common
PIPRA is purchased by hospitals and practices and applied free of charge in the preoperative checkup of a patient.
Possible Side Effects
Common
No known side effects.
Regulatory Compliance
Common
The PIPRA algorithm is certified as a Class IIa under MDR in Europe, indicating compliance with European medical device regulations. Ethical compliance is suggested through this certification and the study's adherence to international guidelines for delirium prevention
Level of Evidence
Common
Level of evidence for AI development The PIPRA algorithm's level of evidence can be assessed based on the systematic review included in its development and validation, as well as the thoroughness of the analysis methods. Study Quality and Data Sources: An Individual Patient Data (IPD) meta-analysis was performed using data from 21 studies involving 8,528 patients. These studies were rigorously selected and included a wide range of perioperative factors. Most included studies were cohort studies from diverse geographical regions, including Europe, Asia, North America, Australia, and South America, enhancing the generalizability of the findings . Level of evidence for AI validation The algorithm was validated using both internal and external datasets, with performance metrics such as the area under the receiver operating characteristic curve (AUC) reported. Internal validation showed an AUC of 0.80 (95% CI: 0.77-0.82), while external validation reported an AUC of 0.74 (95% CI: 0.68-0.80) . Multiple imputations were used to handle missing data, and the one-stage IPD meta-analysis approach was employed to account for clustering within studies and enhance the robustness of the findings. Results and Sensitivity Analysis: The probability of experiencing POD was calculated using a multilevel mixed-effects logistic regression model. The results were consistent across various sensitivity analyses, indicating the stability and reliability of the model.
EQUAL CARE® Certifications
EQUAL CARE® Certification
AI-Supported Diagnostic / Treatment
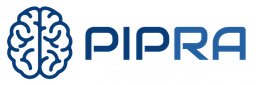
PIPRA AG